I’m going to let you in on a little secret: Without power grid modeling tools, the transition to clean electricity would be an absolute mess.
Just imagine if grid planners had to guess how many renewables are required to reduce emissions or how much capacity is needed to ensure grid reliability. Imagine grid planners shrugging their shoulders and saying, “Let’s just try it and see what happens!” Surely that would not end well.
Luckily, we don’t have to resort to guesswork because we have sophisticated grid modeling tools that help guide the transition to clean electricity. Collectively, these tools can show how to reduce global warming emissions while keeping costs down and the lights on. Grid modeling tools have a significant real-world impact because utilities, grid operators, regulators and policymakers rely on these tools to make investment and policy decisions.
Grid modeling tools come in different flavors. There are many different grid models designed to answer different types of questions. And while there’s a wide array of models out there, they can generally be categorized into four different types. I’ll walk through each of the four types of grid models, discuss how they work and the questions they answer, and give some examples.
Are you ready for my wonkiest blog post yet? Here we go!
Capacity Expansion Modeling
Capacity expansion models are the ones that get all the glory these days because utilities, grid operators and regulators use them to make investment decisions. These models are essentially designed to determine what resources need to be added to the grid to meet certain goals, such as clean energy or emissions reduction goals. So, whenever you see such headlines as “CPUC proposes optimal 2030 system portfolio tripling battery storage, more than doubling solar,” you can be pretty certain that a capacity expansion model was involved.
On a more technical level, capacity expansion models involve complex optimization. The goal is to minimize grid costs while meeting certain objectives (called “constraints” in the modeling), such as reducing global warming emissions, meeting renewable and clean electricity standards, and ensuring grid reliability. The optimization selects the mix of new grid resources that satisfies all the constraints at the lowest cost.
With that in mind, capacity expansion models are well-suited to answer such questions as “How much and what types of renewables should a utility build to meet its renewable electricity standard obligations at the least cost?”
As you can imagine, this type of grid modeling gets really complicated really fast, so modelers often make simplifying assumptions so that they don’t have to use massive supercomputers to run their models. For example, the California Public Utilities Commission uses a capacity expansion model called RESOLVE, and it’s simplified by simulating only 37 representative days per year by combining regions to reduce the complexity of the transmission topology and by “linearizing” power plant dispatch.
Here are a couple examples of recent UCS studies that have used capacity expansion models:
- Federal Clean Energy Tax Credits utilized the ReEDS model to evaluate several federal tax credit policies, assessing their impact on the buildout of renewable energy resources and power sector global warming emissions.
- Countdown to Shutdown used the RESOLVE model to determine what clean resources California needs to build to replace Diablo Canyon—California’s last nuclear power plant—before it shuts down in 2025.
- Let Communities Choose used the HOMER model to do a smaller scale optimization, finding the lowest-cost mix of local clean resources that meets Highland Park, Michigan’s annual electricity demand.
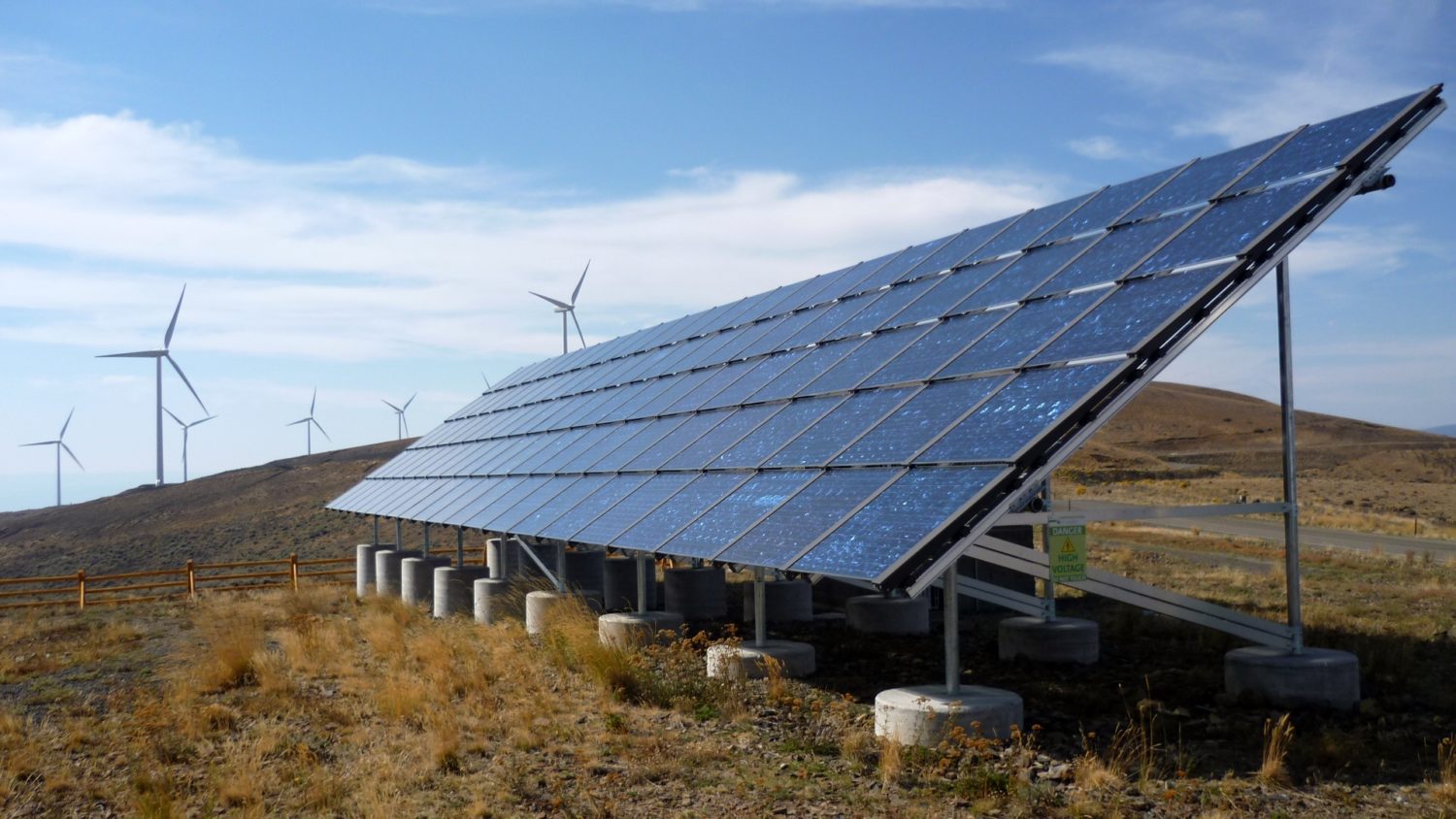
Capacity expansion modeling helps determine how many clean resources, such as solar and wind, need to be added to the grid to meet clean energy goals. (Photo: M. Ewert/Flickr)
Production Cost Modeling
Production cost modeling is used to conduct detailed simulations of grid operations and costs. Production cost modeling and capacity expansion modeling are similar in that they both use optimization to find the least-cost dispatch of grid resources. However, whereas capacity expansion modeling selects new resources to add to the grid over a range of future years, production cost modeling uses one static set of resources on the grid and usually examines a snapshot in time (e.g., a single year). This narrower focus allows grid modelers to do much more detailed analysis of grid operations before the optimization becomes too complex.
Many of the simplifications often made in capacity expansion modeling are not used in production cost modeling. This type of modeling almost always examines all 8,760 hours in a year (if not sub-hourly grid operations), it usually includes much more detailed transmission topology, and it more accurately simulates the intricacies of power plant dispatch. (If you want to get even wonkier, ask me about the joys of accurately modeling power plant heat rate curves.)
Because of its detail-oriented nature, production cost modeling is best suited to answer such questions as “How much will fossil-fuel power plants operate and how much emissions will they produce?,” “How much will it cost to operate the power system next year?,” and “How much solar curtailment will occur on a grid with 50-percent renewables?”
Here are a couple examples of UCS studies that have used production cost modeling tools:
- Used, But How Useful? used PLEXOS to study coal power plant operations across 15 states, finding that coal plants operated even when cheaper and cleaner resources were available, costing ratepayers an extra $350 million in 2018.
- Achieving 50-Percent Renewable Electricity in California also used PLEXOS to study grid operations on a 50-percent renewable grid and specifically examined strategies to reduce solar power curtailment, which in turn would reduce global warming emissions and costs.
Probabilistic Modeling
While some folks might view probabilistic modeling as a subset of production cost modeling, I don’t think that does it justice. Probabilistic modeling, also called stochastic modeling, is playing an increasingly critical role in ensuring grid reliability through the clean energy transition, and this type of modeling is sufficiently different that it warrants its own section in this blog. (Full disclosure: I used to work at Ascend Analytics, a company that has its roots in stochastic grid modeling, so maybe I’m biased!)
At its core, each simulation in probabilistic modeling is essentially just production cost modeling in that the goal is still to optimize grid dispatch to minimize costs. However, the key difference is that probabilistic modeling includes hundreds or even thousands of simulations, so it’s like running a production cost model over and over, changing certain assumptions in each simulation.
From one simulation to the next, the variables that change are usually weather conditions, electricity demand, renewable energy production, and power plant forced outages. There are two keys to developing accurate assumptions for probabilistic modeling. The first is to utilize a wide range of scenarios that accurately reflect the probability that certain events will occur, and the second is to preserve the real-world relationships between the variables that change from one simulation to the next. For example, in the California Independent System Operator territory, where the historical peak load is 50,270 megawatts (MW), you wouldn’t want half of your probabilistic simulations to have a peak load of 75,000 MW, because that’s just not realistic. Likewise, on days with very high electricity demand, which almost always occurs on hot summer days in California, you wouldn’t want your solar output to be abnormally low, since that’s not realistic for a hot sunny day.
The standard practice for developing probabilistic grid modeling assumptions is to use historical data. This data tells you the historical probabilities of certain events occurring on the grid (e.g., days with very high load), and it also provides information about the correlation between variables (e.g., the relationship between load and solar production). However, there’s this problem called climate change that is throwing a wrench into the mix. Climate change is already triggering hotter and more extreme weather, and it’ll only get worse. Simply using historical data for probabilistic grid modeling just isn’t going to cut it anymore.
Now, you may be wondering, what’s the point of probabilistic grid modeling? Despite its complications, this type of modeling is critical for ensuring grid reliability. When you run hundreds or thousands of simulations, you can use statistics to calculate reliability metrics, such as loss of load expectation (LOLE), and expected unserved energy, which tell you whether or not you’ll meet the industry-wide grid reliability standard. Probabilistic modeling can also be used to determine the reliability contribution of certain types of resources via effective load carrying capability (ELCC) calculations, which I’ve previously blogged about in relation to renewables and energy storage. Finally, utilities also use this type of modeling to calculate financial risk metrics, such as value at risk.
Here are a few recent examples of probabilistic modeling studies:
- The California Public Utilities Commission and the consulting firm Astrapé used SERVM to conduct a combined LOLE/ELCC study to reassess resource adequacy compliance requirements for 2024 and to calculate ELCC values for solar, wind, storage and hybrid resources.
- The consulting firm E3 used RECAP to assess resource adequacy in the Desert Southwest region to determine if additional resources are needed to ensure grid reliability. In the course of the study, it calculated both LOLE and ELCC metrics.
- I’d be remiss not to mention my old friend PowerSimm, Ascend Analytics’ flagship product, and the model I helped utilities use to analyze financial risks.
Network Reliability Modeling
Lastly, network reliability modeling is used to do very detailed simulations of the transmission network to make sure the system functions properly and can handle contingencies. This type of modeling is typically conducted on a much shorter timescale (only seconds or minutes), and it can be used to examine grid reliability factors such as voltage stability and frequency stability that can’t be analyzed with the previously mentioned modeling tools.
One real-world example is that the California Independent System Operator (CAISO) uses this type of modeling to conduct power flow analysis and develop local resource adequacy requirements, a critical element of maintaining grid reliability in California. The idea behind this type of analysis is that you can examine contingencies, such as a power plant or transmission line tripping offline, and determine if the grid will fail as a result.
One of the most difficult aspects of the clean energy transition has been figuring out how to reduce reliance on fossil-fuel power plants needed for local grid reliability, and this type of modeling can help. Here are two examples:
- When NRG proposed building a new gas power plant, the Puente Power Project on California’s coast, the CAISO used this type of modeling to conduct a power flow analysis to assess alternative portfolios of clean resources. Ultimately, it found that the gas power plant is not needed.
- UCS’s Soot to Solar analysis examined the reliability impact of shutting down the coal- and oil-powered Waukegan Generating Station in northern Illinois. UCS retained the engineering firm PowerGEM to conduct power flow modeling, finding that the Waukegan plant could be shut down if it were replaced with 100 MW at the same location.

Power flow modeling helped identify reliable clean energy alternatives to the Puente Power Project, which would have replaced the Mandalay Generating Station, pictured here. (Photo: Jeremy Wheaton/Flickr)
Three Important Caveats
I should mention that many grid modeling tools can be used for multiple types of grid modeling. For instance, I mentioned the PLEXOS model that UCS has used to do production cost modeling, but the CAISO also has used PLEXOS for probabilistic grid modeling, and the tool can be used for capacity expansion modeling as well. Many of the grid models I mentioned in this post (e.g., SERVM and PowerSimm) are in a similar boat. They also can perform multiple types of grid modeling.
Second, it’s also important to recognize that different types of grid models are better suited to answer certain types of questions. For example, you wouldn’t choose a capacity expansion model to do a deep-dive study on grid reliability. You would likely choose a probabilistic model or a network reliability model, depending on your exact research question. You should always choose a model based on your research question, not the other way around.
Last, but certainly not least, the results from these models are only as good as the assumptions that go into them. There’s a common saying that sums this up nicely: garbage in, garbage out. Depending on the inputs used, the same model can produce very different results, and that’s why it is vitally important to use well-vetted assumptions from credible, independent sources. For example, if you overestimate the cost of renewables, your modeling might end up lowballing the buildout of renewable resources. Or, if you go into a study with biased assumptions about the reliability benefits of certain types of resources, your results are going to show it. Modeling results are only as good as the inputs.
Grid Modeling for the Win
With the transition to clean energy well underway, grid modeling tools help guide the way. They aid grid planners to select new resources, keep costs down, ensure grid reliability, and so much more. If you’re as pumped about grid modeling as I am and want to learn even more, I highly recommend this excellent Department of Energy PowerPoint presentation. Otherwise, congrats on making it through this post!